- Sat 26 April 2025
- Experiments
- akuz
🎛️ Co-Designing a Sparse Music Codec with ChatGPT o3 in One Day — My Mini Pied Piper
For years I’ve wanted to build a super-dense electronic-music compressor: keep only the loops and phase cues that really matter, then re-synthesise the track perfectly. Evenings and weekends, however, were never long enough to design the model, write the maths, and wrangle PyTorch. Recently I opened ChatGPT running the …
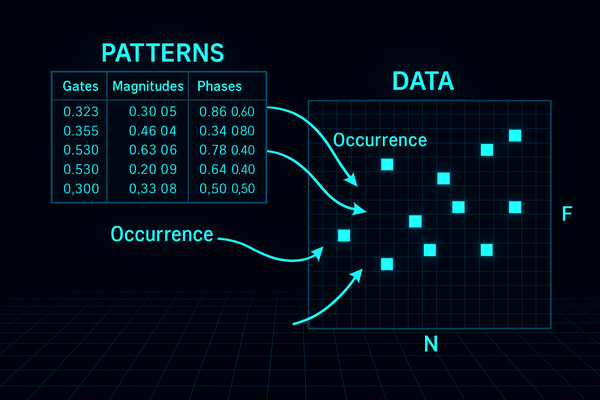